admin2023-09-27T06:29:01+00:00
Table of Contents
ToggleTake yourself to around 20 years ago, remember photographs or videos of that era. They lack details in most of them because of “Image/video quality”. Yes at that time little we knew about the term “Noise” all we knew was images are either fine or blurred. It was a major issue then and the same as it is now but thanks to the image processing field we have conquered our challenges.
If we talk about real-time graphics, either still or motion, all of them are subjected to noise. Noise is usually referred to as any interference generated randoms in those graphics. This unwanted interference can be the change in colors of images and add brightness. Pixels changings etc.
When we specifically talk about video graphics, this noise is caused by low lighting conditions. Low light is terrible luck for the less sensitive cameras. A lot of information is lost in the absence of sufficient light. Therefore these cameras are not suitable be used in security surveillance systems.
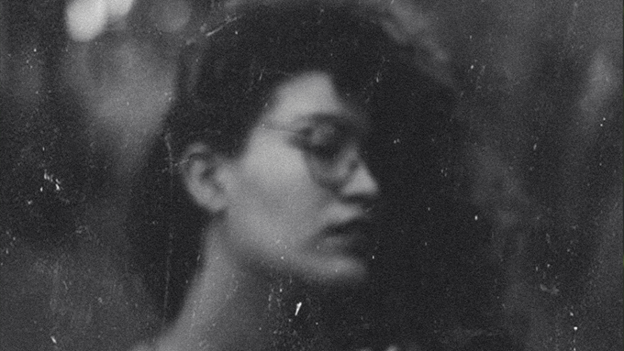
Image noise has quite an impact on the brightness of the captured photos, in addition to making it difficult or impossible to identify people and vehicle license plates. It may also impact how well the monitoring system works in poorly lit commercial and office spaces. Where identification is the primary priority, noise reduction is required everywhere.
Security cameras, like many other environmental sensors, gather data from the surrounding environment and are therefore subjected to the threat of all-natural factors, including called noise. All the footage captured by a security camera may have errors and corrupted information, which is quite a concern when we talk about “Security cameras,” & if noise is layering the information, the camera is not serving its purpose because it is unable to get rid of the environmental noise.
Given that a camera’s ability to record noise-free video depends on its sensor, this is a challenging control to maintain. However, experts have developed a number of methods to lessen noise. These methods are referred to as “Digital Noise Reduction” techniques, and that is what this article will cover.
What Exactly is Noise?
Noise is, to put it simply, undesired information. Noise in cameras degrades video and, in the worst situations, can obliterate crucial details in a frame. (In this instance, a frame is an image that was taken with a camera.
Because sensors are dumb instruments, the noise will always be present in images. However, noise is more problematic for footage captured in poorly lit areas. As a result, if you snap a shot in a dimly lit location, you can notice that the Image (or some areas of it) has a few small, irregularly colored pixels or groups of pixels. These are how noise in an image is represented visually.
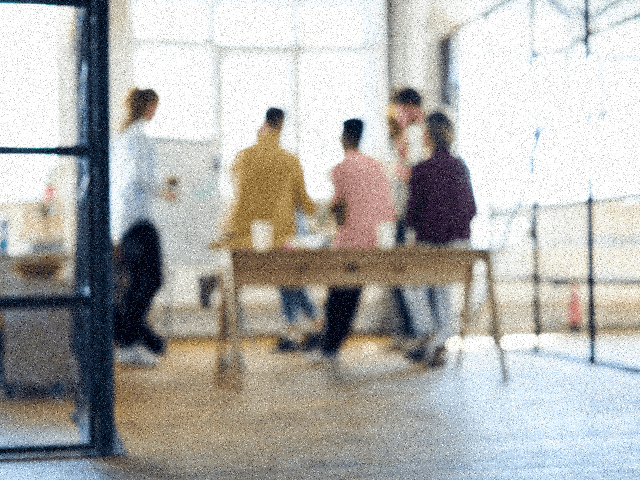
Noise is a result of flaws in the camera’s light sensor. Although cameras have advanced significantly, their fundamental purpose still depends on gathering enough light from the surroundings. Therefore, the better the image, the more light it can muster. However, we cannot allow the sensor extra time to complete a single picture since security cameras must capture video in real-time (preferably 24 or 30 frames per second). As a result, the camera will produce images with some noise when it is dark outside.
Explaining Digital Noise Reduction (DNR) In Security Cameras
2D and 3D Dynamic Noise Reduction is a widely used image processing technique to decrease noise from the image. You may have seen grainy pictures on the screen, which destroy image quality and make images hard to see. 2D/3D DNR (Digital Noise Reduction) technology uses different algorithms and makes the image high quality.
What Types of Digital Noise Reduction are there?
There are two techniques that are being used to overcome the noise that is recorded in video images.
- 2D DNR (Also known as 2D Noise Reduction)
- 3D DNR (Also known as 3D Noise Reduction)
What is 2D DNR?
2D DNR is one of the most straightforward techniques to remove noise from a video image. The 2D noise reduction does remove noise from images, but it is limited to low-resolution images. 2D DNR failed to provide results in HD / HQ images where the Image contains a lot of movement & minor detailings.
How does 2D DNR Work?
2D DNR is also known as a “Temporal Noise Reduction” technique. Its works by comparing the frame of a video image.
The pixels of each frame are compared with the pixels of the next frame. So by comparing the intensity and colors, the noise is detected & hence removed. It develops algorithms by comparing pixels where the noise is detected in patterns.
After the patterns are identified, the software assigns an average intensity & color to the pixel which was subjected to noise. And the Image is cleared out.
From the above description of the algorithm working for 2D DNR, you may have understood the working principle. But this technique works fine only for the static Image, with no movement. The images with the movement have more information, and thus comparing frames can cause picture blurriness.
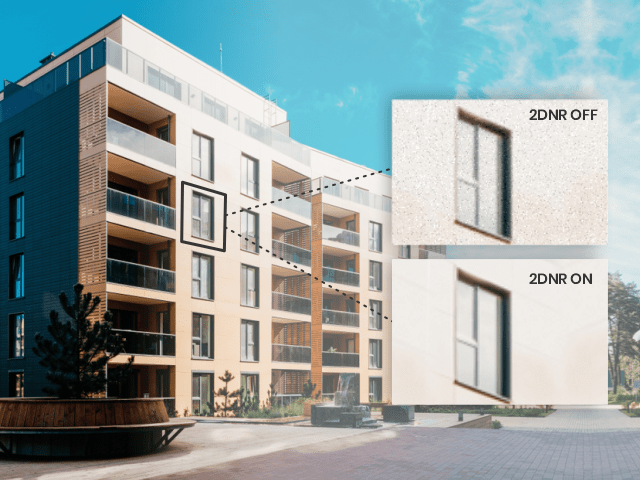
The algorithm will try its best to determine which hue or intensity is the most natural for each pixel, which may result in motion blur even though it may operate in a static environment with no movement.
Additionally, because of the limitations of the system’s computational power, it is not possible to execute these algorithms at higher resolutions and deliver them in real time. They are able to process, but it takes a long time.
What is 3D DNR?
The 3D DNR is an advanced technique of noise reduction. The 3D DNR is pretty distinct. Although it does contain a few minor temporal aspects, it is referred to be a “Spatial Noise Reduction” technique since it compares and analyzes pixels in three dimensions. (First, an image’s two dimensions, then its third dimension when comparing pixels across frames.)
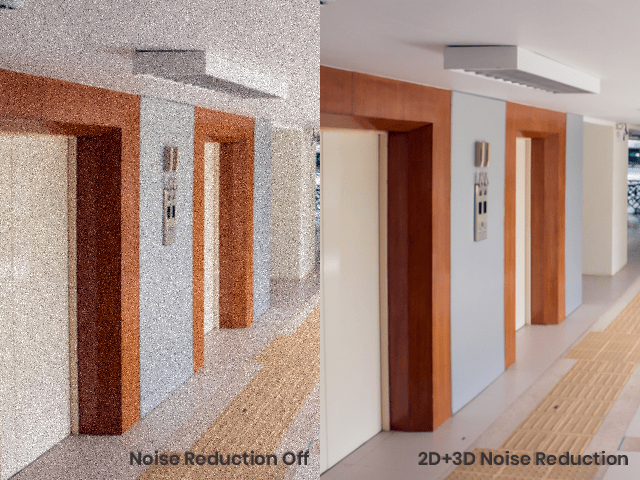
The different algorithms allow 3D DNR to be handled significantly more quickly than 2D DNR. As a result, this technique is preferred to utilize in images/video frames with higher resolution.
How does 3D DNR Work?
The system compares the pixels in one frame while performing 3D DNR. It attempts to remove the grainy appearance of a single frame by employing methods like “median filters” and “averaging filters” to reduce noise within a single frame. In order to detect any motion in the video, it then compares the pixels frame by frame. (Motion is detected by taking into account variations in brightness/color on the same pixel coordinate across two (or more) separate frames.)
Difference Between 2D and 3D DNR
2D DNR Technology works on the frame-by-frame analysis of the footage. 2D noise reduction uses the algorithm to identify the “bad” pixels with noise and correct them.
3D DNR is very similar to 2DNR; the initial processing is the same. But 3DNR additionally analyzes and compares the differences between consecutive frames to adjust pixels and improve fidelity.
3D DNR Technology is a better choice for motion graphics. It’s more effective at reducing noise without creating motion blur, whereas 2D DNR is better at decoding and removing the noise from static frames.
Advantages of 2D 3D DNR in CCTV Camera Surveillance
It can be incredibly annoying to hear background noise in the security camera footage. They may impact sophisticated processing algorithms like motion detection, facial recognition, number plate identification, etc., making it more difficult to tell what is happening.
Additionally, if the system doesn’t handle the noise and optimize frames, collecting RAW files may put a strain on the storage system. After denoising, the images can be shrunk because they are much more straightforward for humans and machines to understand.
What is recommended here is to adjust the environmental light either naturally or artificially. This will automatically compress noise in any image. But the limitation is that “Light is out of our control,” but still, we say prevention is better than cure.
However, it’s not always under our control. We admit that, therefore, a camera with better DNR techniques is an essential need in any security system camera: a necessity for better image quality.
2D+3D Integrated Approach
Both noise reduction approaches, 2DNR & 3DNR, have pros and cons. It’s better to combine both technologies. Some cameras may not apply them simultaneously but offer a choice for the user to switch between 2D and 3D.
The 2D+3D noise reduction integrated approach is more likely applicable in high-definition images and graphics, i.e., engineering and computer for analysis or visualization of high-quality models. In this approach, 2D data is from 2D images or photographs, while 3D data is from 3D scans or models. The 2D and 3D data are then combined and processed to reduce noise and enhance the overall quality of the image or model.
Impact of Brightness on Image Noise
As we previously discussed, when a video is recorded in a low-light setting, noise is more noticeable in the photos. Therefore, it is acceptable to say that the brightness of a scene can influence how visible noise appears in a frame or Image.
Like many other sensors, cameras are susceptible to noise, and the measurements they take will never be a precise representation of reality. However, there will always be some level of noise in whatever Image that a camera takes. To combat this noise or to achieve a higher SNR, more information must be gathered (SNR: Signal to Noise Ratio)
Brightness is a significant factor in getting more signals to overwhelming noise. The camera is excellent at absorbing light to deliver a crisper image in brighter and better-lit environments. In a nutshell, when a scene is recorded in low light, the camera lacks sufficient information, and noise builds up.
Therefore, while noise reduction methods used in post-processing, including 2D and 3D DNR, can reduce noise to some amount, it is always preferable to improve the brightness before taking a video.
Impacts on Storage
The storage does not seem to be affected immediately by noise, apparently. But if the user attempts to adjust the image/video files’ resolution, it may have an impact on the file size. Storage is indirectly related to noise.
The complexity of the DNR algorithms is the source of this since some cameras and systems lack the processing power necessary to compute these algorithms in real time with little latency. Some systems may choose to reduce the resolution of a frame or Image as a result to lessen the visibility of the noise.
when the resolution of the Image is decreased, there are fewer pixels to process. As a result, the system can compute the DNR algorithms more quickly and use less hard drive space & vice versa.
Noise doesn’t therefore directly affect storage; rather, it only does so if the user or system decides to change the resolution of the frames or images.
Impact on Motion Detection
Motion detection algorithms can be impacted by noise. As we previously explained, the 2D DNR algorithm dislikes continuous motion in the footage since it results in motion blur in the final footage due to its post-processing method.
Fortunately, motion detection is not hampered by motion blur. As long as the motion blur effect does not blend in with the surroundings, the system will always detect motion. However, motion blur can have drawbacks, making it challenging to detect crucial details like faces and license plates due to the hazy appearance of the object in the frame. Therefore, it’s recommended to reduce the motion blur effect by using 3D DNR methods or comparable post-processing techniques for motion detection and other requirements of information gathering.
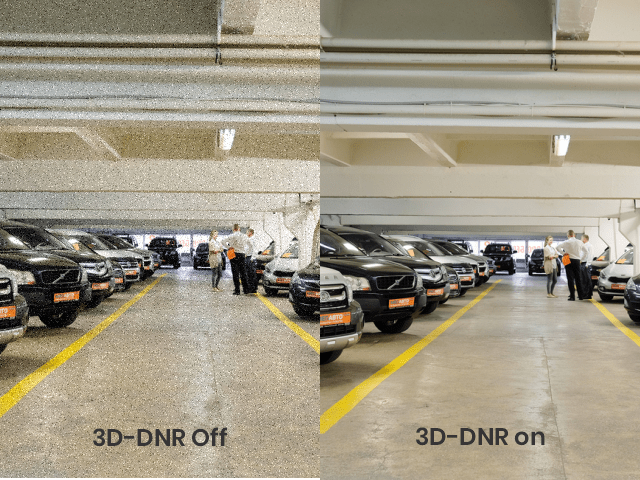
Noise Reduction Approaches
Since image noise affects image quality, reducing noise while preserving salient details is a top priority for security camera manufacturers. Increasing the illumination may allow your camera to capture noise-free images, but sometimes ideal lighting is impossible for many video surveillance applications. Various hardware and software-based noise reduction technologies have developed in recent years. They are devoted to reducing noise in the video captured by CMOS and CCD image sensors.
Noise Reduction Reduces False Motion Alerts
Noise reduction helps to filter out non-movement-based disturbances that can trigger motion detection sensors, such as wind, rain, and tree branches. With noise reduction, the camera’s sensors can better distinguish actual motion events from false alarms caused by environmental factors.
Without a noise reduction, any camera can confuse objects and falsely detect motion because of the grainy images. Every camera comes up with a motion alerts sensor, or you can set up one. The motion alert notifications can be emails, mobile messages, alarms, snapshots, or push notifications on your mobile phone through the app.
Temporary Noise Reduction vs Spatial Noise Reduction
According to the information we have discussed till now, 2D DNR is a temporal-based technique, whereas 3D DNR, as mentioned earlier, is a spatial-temporal combination with the major algorithms executed in the spatial domain.
Both approaches have their own set of problems despite the fact that they can both reduce noise. It’s not difficult to set up the 2D DNR (also known as the temporal noise reduction approach). The processing of larger photos, however, takes an unacceptably long time and results in a motion blur afterward. It cannot be used to denoise a single image and instead requires many frames for noise reduction.
The implementation of spatial noise reduction techniques is challenging and calls for advanced processing computational infrastructure. Their algorithms, however, are quicker and perfectly compatible with cameras that have more megapixels. Additionally, they may denoise a single frame or several frames if necessary without producing a motion blur impression.
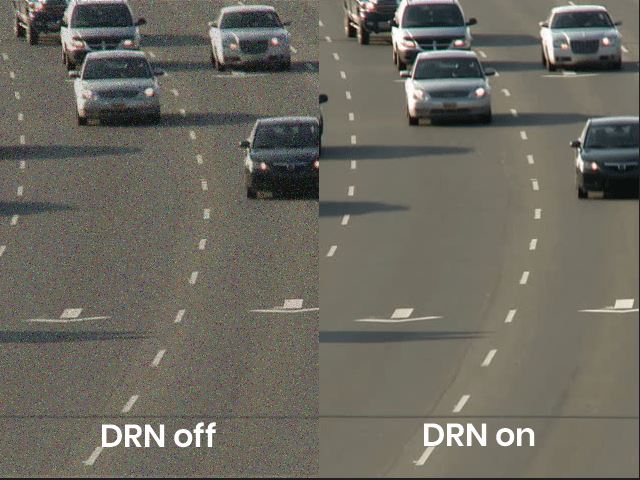
Benefits of Noise Reduction
Digital Noise Reduction is very important in cameras because it is related to better image quality. 2D & 3D DNR increase the video quality. 2D & 3D DNR removes the grainy spots from images and makes them more visible. CCTVs are specifically for security purposes, and in case of a mishap, it is essential to identify a person or vehicle from CCTV footage. You can only extract information from such images if the footage is clear.
Conclusion
CCTV security cameras need to utilize digital noise reduction techniques because they reduce the grainy appearance that results from recording video in low light. By reducing noise, the image becomes clearer and is easier for humans and machines to comprehend. The 2D DNR and the 3D DNR are the two denoising algorithms used by the majority of CCTV cameras. While the 2D DNR algorithm is straightforward and can be found on many older, less expensive systems, the 3D DNR algorithm is more popular since it can denoise images with higher resolutions without introducing motion blur.
The majority of high-end cameras integrate both methods for better interference reduction. While 2D-DNR technology produces superior results for moving objects and is employed in places where motion is monitored, 3D-DNR technology is most frequently used in connection to static sections of the monitored space. The best noise reduction results come from an algorithm that allows for customizable integration of the two technologies, which also produces clear images even in low light.
In addition to having a negative impact on image quality, noise shortens recording times, increases video stream size, and can result in false alarms. It also increases the amount of disc space needed to store images. Therefore, the noise reduction function is essential for CCTV cameras working in erratic and low lighting settings in order to remove interferences.
FAQs
2D and 3D Dynamic Noise Reduction are powerful image processing techniques to remove noise from images. Digital Noise Reduction plays a significant role in HD footage, also known as 4k video. 2DNR + 3DNR is used for such footage to remove the noise and make the image high quality.
2D DNR is also called DNR 2. It is a technology in image processing through which the noise is removed from the static images to make images more vivid.
WDR is Wide Dynamic Range (WDR) technology used in black and white or high contrast images to improve image quality by enhancing the light and dark pixels in the field of view. WDR uses sensors to enable the camera to capture every detail clearly in both the poorly and strongly illuminated areas of the video.
DWDR is Digital Wide Dynamic Range used in CCTV cameras to adjust the light in the footage. DWDR technology identifies the less dark pixels for under-illuminated or dark images and adjusts the light accordingly. If the image is over-illuminated, DWDR identifies the dark pixels and modifies them, making image details easily readable.
DWDR uses image processing algorithms to identify and brighten up dark pixels and vice versa digitally. WDR analyzes individual pixels of the image and calculates exposure accordingly. In comparison, DWDR uses the digital signal processing chip, also known as DSP Chip, to remove noise from high-contrast images.
Here are the general steps to change the DNR setting on most cameras:
Access the camera’s menu: Depending on the camera, you may need to press a specific button or use a touchscreen to access the menu.
- Open the menu, then go to settings related to the camera’s image quality or picture.
- Within the image quality or picture settings, you should be able to find a setting for Digital Noise Reduction (DNR). The specific wording of the setting may vary.
- Once you’ve found the DNR setting, you can adjust it to your preference. Some cameras may have multiple levels of DNR, while others may only have an “on/off” option.
- Once you’ve made your desired changes, save the settings before exiting the menu.
It’s important to note that adjusting the DNR setting may affect the overall image quality of your photos or videos. While reducing digital noise can help create a cleaner image, it can also reduce the sharpness and detail in your photos. Therefore, it’s essential to experiment with different settings to find the right balance between noise reduction and image quality.